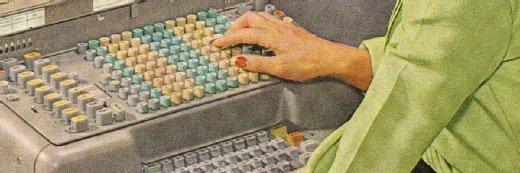
Getty Images
Referential Algorithms Boost Patient Matching Accuracy
Referential algorithms include additional health data sources to support patient matching by building a more complete profile of each patient.
Referential patient matching algorithms demonstrated greater accuracy than the traditional probabilistic approach, according to a study published in JAMIA.
Probabilistic software implements a weighted similarity algorithm that uses blocking schemes to gather candidates sharing at least a portion of matching fields.
“Candidate matches are scored attribute-by-attribute using a weighted similarity based on discriminating power, summed across matching attributes,” the authors explained.
“The set of attribute data is evaluated using heuristic rules for specific conditions that increase or decrease the likelihood of the match and adjust the weighted score accordingly,” they added. “The system declares the records a match if the final match score exceeds a configurable threshold.”
Referential algorithms are similar to probabilistic software, but they use additional data sources.
The Pew Charitable Trusts defines referential matching as “leverag[ing] data from different sources to build a more complete profile of each patient that includes past addresses, common name spellings for individuals, and other demographic data that changes over time.”
Using a manually reviewed 30,000 record reference dataset derived from a health information exchange (HIE) with more than 47 million patient registrations, researchers assessed matching accuracy for referential and probabilistic matching algorithms.
The study authors evaluated matching performance using sensitivity, positive predictive value (PPV), and F-score (the harmonic mean of sensitivity and PPV), which provides an overall measure of matching accuracy.
The study authors found that referential matching demonstrated greater accuracy than the more traditional probabilistic approach.
The probabilistic algorithm exhibited an F-score of 0.7778, while the referential algorithm exhibited an F-score value of 0.9663.
“Record pairs with referential match and probabilistic nonmatch statuses contained various combinations of changing name, address, phone number, or missing values,” the authors wrote.
“These reflect cases where patients appeared to change location, use both nicknames and given names, change or use multiple phone numbers, have typographical or recording errors in any of these values, or have incomplete data,” they said.
Referential sources more completely captured these shifting demographic combinations over time to identify relations between seemingly distinct identities, which significantly increased patient matching sensitivity, the researchers noted.
“Pragmatically, the findings from this study provide transparency and can be meaningful to those making decisions regarding identity matching solutions,” they wrote. “Consequently, health IT policymakers, including ONC, should explore strategies to expand the evidence base for real-world matching performance.”
The study has two main limitations. First, reference data quality influences referential matching performance.
“Thus, referential matching systems using reference data with differing coverage for population subgroups such as children and the homeless or reference data with error rates varying from the system evaluated may yield different results,” the authors pointed out.
Additionally, while the data included represented a broad spectrum of healthcare settings, the analysis used data specific to Indiana health systems. Results may vary in environments with differing demographic data characteristics.